How Predictive Analytics Is Shaping Tomorrow's Industries
Thursday, February 13, 2025
SHARE
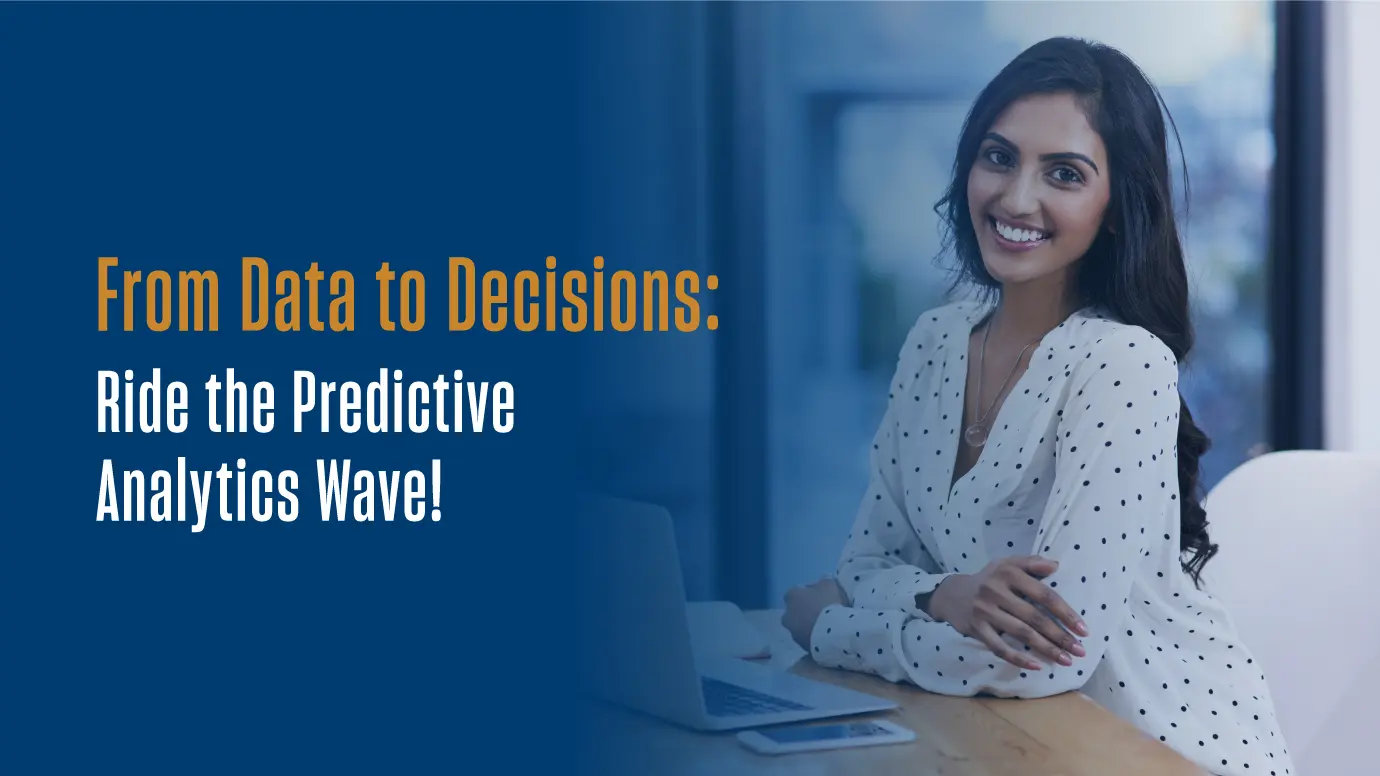
Explore the future of predictive analytics and its role in shaping industries by 2025. Discover how an MS in Applied Data Science prepares you for a career in this high-demand field.
Why Predictive Analytics Is Becoming a Game-Changer for Industries?
Imagine you're a business leader who is looking for a competitive edge in a data-driven market today. You're sitting on a wealth of customer data but struggling to make it actionable. Sound familiar?
This problem is common across many industries: companies may have ample data but very few tools to predict valuable customer behavior, model sales, and optimize operations.
This is where predictive analytics comes into play, a powerful tool that puts the ability to forecast future trends, patterns, and data-driven intelligence at the fingers of decision-makers, enabling more proactive data-centric decisions overall.
Predictive analytics will soon be essential for a wide range of industries such as finance, healthcare, retail, and logistics. So, what is the reason for this growth explosion, and what can professionals do to ensure the positioning to flow with this opportunity wave?
Let’s explore the future scope of predictive analytics, its growing applications, and how an MS in Applied Data Science can prepare you to lead in this dynamic field.
Rising Demand for Predictive Analysis
Predictive analytics can be derived from a variety of data models depending on the type of data available. Organizational factors come into play when choosing which model to use, such as the resources it will take to create the model and how accurate it must be.
Some of the Models and Techniques are:
Time series data models: These are useful when temporal or time-stamped data is analyzed to forecast values. They are effective in scenarios such as time-change or seasonality, although not where the data line is minor through time.
Regression models: Often used for cases like stock returns or house price prediction, regression models are useful when you want to develop a strong relationship between two entities. These models can sometimes provide incorrect data, especially when there are too many variables.
Gradient boosting models: Instead of constructing a single strong model, gradient boosting consists of several weaker models that when put together are strong. It is a good approach for non-linear data prediction, but the drawback is that the models have to be fine-tuned properly and consistently.
Random forest: Like gradient boosting, random forest models are built on a combination of simpler models, primarily decision trees. That means by individually analyzing each tree, you can end up with a final prediction from the entire forest.
Clustering Models: Clustering is mostly used for putting data points in a group, but, it can also be utilized as input for prediction models. The clustering models are useful when we need to discover hidden relationships or clusters in the data but need a mechanism to define how similar two data points are.
Future Scope: Why Predictive Analytics Will Boom by 2025
Predictive analytics is not confined to industries; instead, it spans sectors and develops numerous career pathways.
Healthcare: Hospitals are turning to predictive analytics to improve patient care while minimizing costs. For example, Predictive models can identify a patient's risk of chronic conditions so that an intervention can be issued immediately.
Finance: Banks and insurance companies have already made big investments in predictive analytics as a means to reduce risks or erratic behavior of clients. Individuals qualified with a master's in applied data science are ready to create algorithms to determine fraud and assess credit risk.
Retail and E-commerce: Predictive analytics have played a crucial role in improving customer experience and optimizing supply chains for many years, from Amazon's recommendation algorithms to Walmart's inventory forecasts.
Several factors point to a continued expansion in predictive analytics, making it a crucial field for those in data science:
- Growing Data Availability: Data generation is expected to be more than 175 zettabytes by 2025, and organizations require data-backed devices to evaluate large amounts of information. Predictive analytics saves the day quite efficiently.
- Advancements in Algorithms and Computing Power: The availability of complex data may call for more advanced machine learning models, such as Deep Learning and the emergence of cloud computing makes it easier to process and integrate complex data.
- Data-Driven Decision-Making: As businesses face pressure to retain their competitive positioning, the need for data-driven insights has increased. Predictive analytics provides actionable insights that are increasingly essential in a saturated market.
Career Opportunities in Predictive Analysis
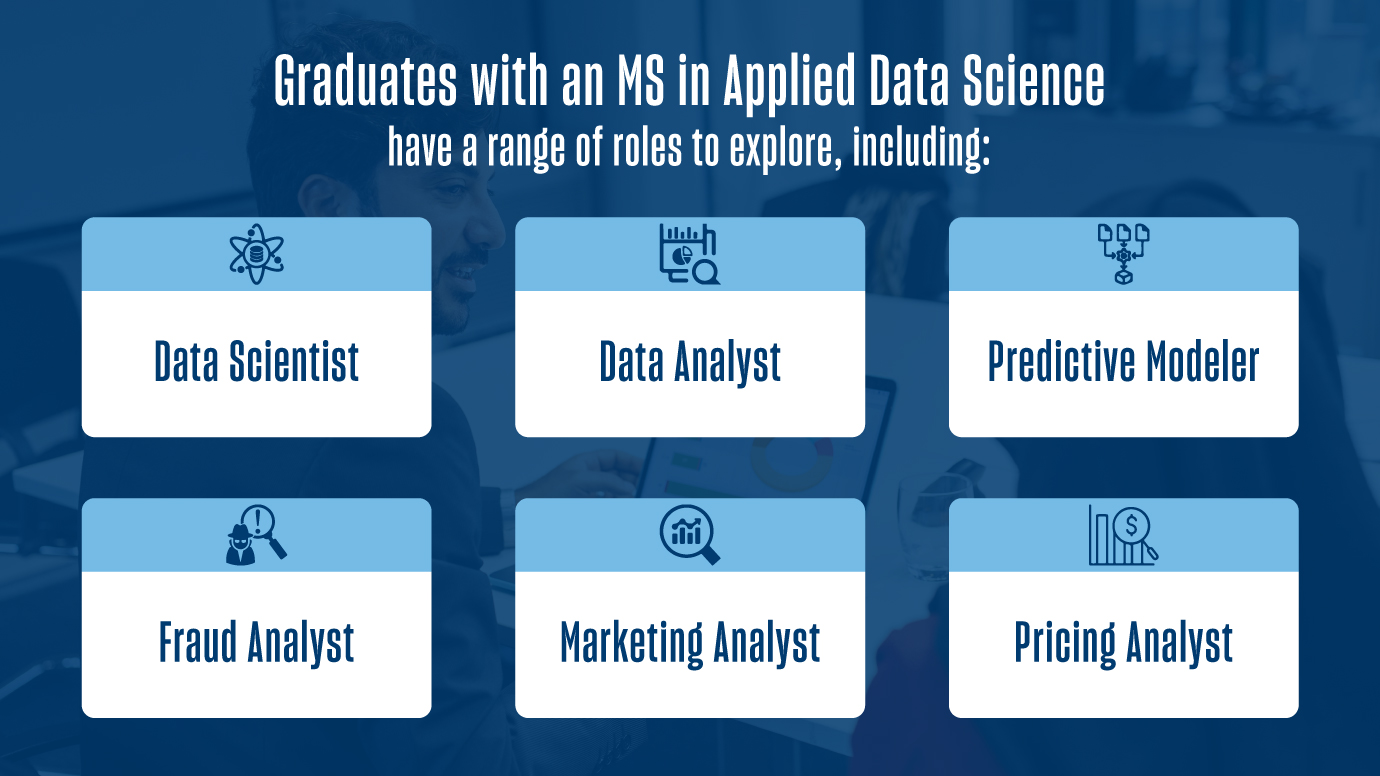
Predictive analysis is a rapidly growing field with an abundance of careers available. With a future full of possibilities, the job market is evolving to prioritize predictive analytics expertise.
Graduates with an MS in Applied Data Science from USD Online have a range of roles to explore, including:
Data Scientist: They analyze data and extract actionable insights using statistical and machine learning techniques. Data scientists are in high demand, with salaries ranging between INR 6 to INR 30 lakhs per year, depending on the level of experience. In some industries like finance and pharmaceuticals, experienced data scientists (10+ years) can go as high as INR 45 lakhs. (Scaler)
Data Analyst: Focused on the analysis of data for trends and patterns generally via SQL, Python, or R. The average salary for a data analyst in India is between INR 3.5 to INR 12 lakhs a year, based on the working knowledge of tools like SQL, Python, R, etc. this is an entry-level role, but can evolve into a critical position. (Glassdoor)
Predictive Modeler: Create models that use the trends and patterns found in historical data to predict future trends and behaviors. Predictive modelers focus on forecasting trends. Their average salary in India is INR 8 lakhs per year with high predictive analytics demand. (Indeed)
Fraud Analyst: They identify and prevent fraud using predictive analytics. Fraud analysts, especially in finance, make around INR 4 Lakhs per year (Indeed). Their expertise in fraud detection and prevention is crucial in sectors like banking, where risks are high.
Marketing Analyst: They analyze customer data to forecast trends and ensure effective marketing strategies. This role pays an average salary of INR 9 lakhs yearly, leveraging customer data for campaign optimization. It’s popular in the e-commerce and digital marketing sectors. (Indeed)
Pricing Analyst: They analyze data to determine the most effective pricing approach for goods and services. The average salary for a Pricing Analyst is ₹7,00,000 per year in India. (Glassdoor)
How does the USD Online MS in Applied Data Science prepare you for future challenges?
For those interested in joining this growing field, earning a master's degree in applied data science online is a strategic step. Such programs equip students with practical skills in data analytics, programming, and machine learning, preparing them to contribute to this data-centric world.
Skill Set: Candidates should possess knowledge of Python, R, SQL, machine learning, and statistical methods.
Practical Experience: Several programs provide students with internships or capstone projects to work on descriptive analytics in real-world environments.
Lifelong Learning: The world of data science is constantly changing, and you need to keep up with the latest and greatest tools and techniques, often quite quickly.
Benefits of USD India Online -
- Equivalent Value and Same Curriculum as On-campus Degree
- Attend the Graduation Ceremony on Campus
- Unlock Your Career Potential with Handshake
- Cutting Edge Tech-focused Curriculum
- Exceptional Career Services
- Unmatched Learning Support and Technology